
Calculating DEMs from a large set of point cloud data on a desktop computer would likely take days to run, and it could crash your computer. Running all of the point cloud data sequentially to calculate the DEMs will require intensive amount of computational power and time. In the methods section we will further explore the approach we used to accomplish processing a single county in a little over an hour with our GalaxyGIS Cluster.Īerial LiDAR data has been collected across much of South Carolina by the SC Department of Natural Resources ( SCDNR) and can be easily downloaded from many sources, such as the the National Oceanic and Atmospheric Administration (NOAA) Data Access Viewer. We obtained point cloud data for several counties in South Carolina and used the RapidLasso LASTools as part of a processing workflow to efficiently generate a DTM and a DSM for an entire county at a time using GalaxyGIS. Therefore, we need to use a type of divide-and-conquer system. In many cases, a standard workstation computer will actually run out of memory and crash when trying to process an entire dataset by itself. Processing this much data could take days to run on a single machine, and that’s a long time to wait for your results. Large areas of interest, such as a county, may be represented by billions of data points which equates to many gigabytes or even hundreds of gigabytes of data. Individual LiDAR files, such as an ASPRS LAS, may contain hundreds of millions of points and be several hundred megabytes to a few gigabytes in size. One of the challenges we face, however, is the sheer amount of data to process. LiDAR point clouds are a rich source of geospatial information and can be converted to high-resolution digital elevation models (DEM), such as digital terrain models (DTM) which represent the bare-ground surface and digital surface models (DSM) which include vegetation and building data. Semantic information management and interoperability seemed to be feasible by the use of the 3DCities Project ESRI tools, since its database structure ensures adding semantic information to the CityEngine model and therefore automatically convert to CityGML for advanced analysis and visualization in different application areas.By Willy Li (CCGT Intern) and Blake Lytle (CCGT UAV and LiDAR Specialist) Final goal was the original model’s semantic enrichment and then its conversion to CityGML format. The model was initially implemented in CityEngine ESRI's software, and then imported to ArcGIS environment. The aim of this paper is to develop an application for managing semantic information of a model generated based on procedural modeling. The scope of CityGML is to reach common terminology, also addressing the imperative need for interoperability and data integration, taking into account the number of available geographic information systems and modeling techniques. CityGML defines geometry and topology for city modeling, also focusing on semantic aspects of 3D city information. Within this context, CityGML was developed as an international standard of the Open Geospatial Consortium (OGC) for 3D city models’ representation and exchange. Nevertheless, these opportunities cannot be totally exploited because of deficiencies in standardization and interoperability in these systems. The existing geographic information systems and the different methods of three-dimensional modeling allow for better management, visualization and dissemination of information.

Despite the progress in the field of geographic information systems and 3D modeling techniques, there is no fully digital 3D cadastre. The complexity of urban environments and the strong need for land administration, intensify the need of using a three-dimensional cadastral system.
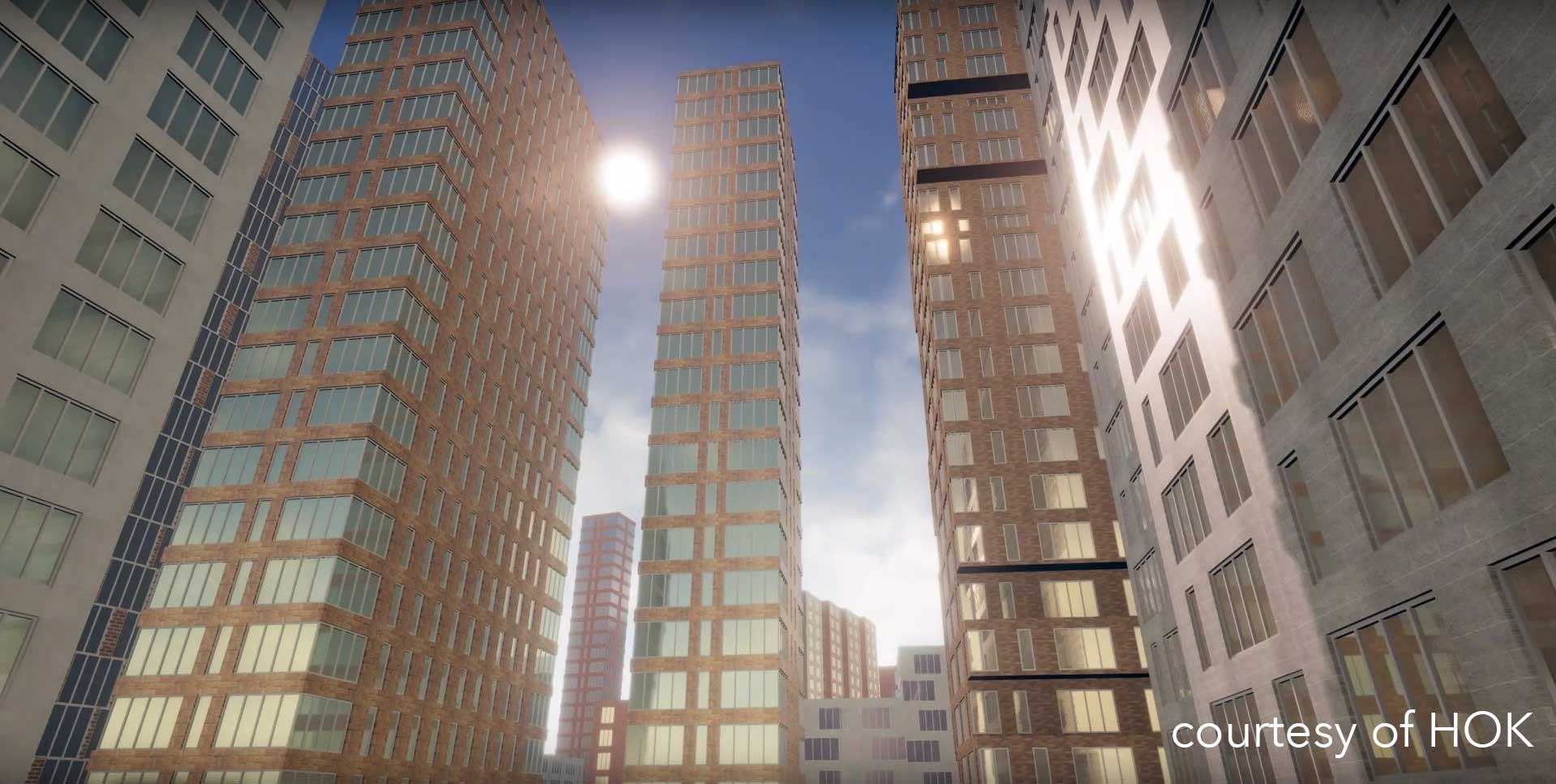
In recent years the development of technology and the lifting of several technical limitations, has brought the third dimension to the fore.
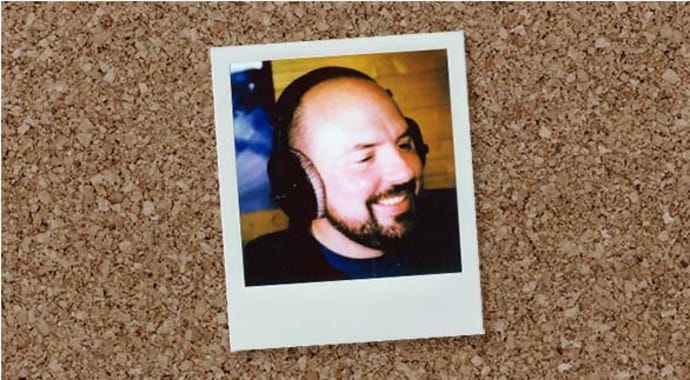
Investigating the capabilities of semantic enrichment of 3D CityEngine data Investigating the capabilities of semantic enrichment of 3D CityEngine data
